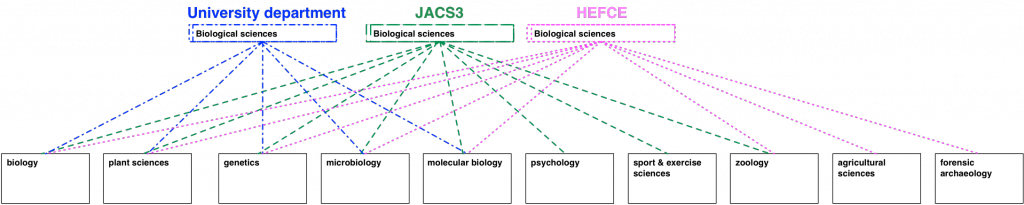
- is the term in JACS3?
- is there evidence of use of the term in HESA data returns?
- is the term’s definition and scope sufficiently clear and comprehensive to allow classification?
- is the term reliably distinguishable from other terms?
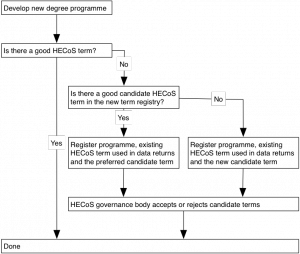
On 17th-18th June, in Bolton, Cetis had their more-or-less annual conference. One of the sessions was Lorna and me, with some help from our friends, discussing LRMI addressing the question “What on Earth Could Justify Another Attempt at Educational Metadata?”
(A contribution to Open Education Week — see note at end.)
Imagine what could happen if we had a really good sets of usable open learning outcomes, across academic subjects, occupations and professions. It would be easy to express and then trace the relationships between any learning outcomes. To start with, it would be easy to find out which higher-level learning outcomes are composed, in a general consensus view, of which lower-level outcomes.
Some examples … In academic study, for example around a more complex topic from calculus, perhaps it would be made clear what other mathematics needs to be mastered first (see this recent example which lists, but does not structure). In management, it would be made clear, for instance, what needs to be mastered in order to be able to advise on intellectual property rights. In medicine, to pluck another example out of the air, it would be clarified what the necessary components of competent dementia care are. Imagine this is all done, and each learning outcome or competence definition, at each level, is given a clear and unambiguous identifier. Further, imagine all these identifiers are in HTTP IRI/URI/URL format, as is envisaged for Linked Data and the Semantic Web. Imagine that putting in the URL into your browser leads you straight to results giving information about that learning outcome. And in time it would become possible to trace not just what is composed of what, but other relationships between outcomes: equivalence, similarity, origin, etc.
Even in the knowledge that current mainstream EPUB readers and applications for managing eBooks will most likely ignore all but the most trivial metadata, we still have use cases that involve more sophisticate metadata. For example we would like to use the LRMI alignment object in schema.org to say that a particular subsection of a book can be useful in the context of a specific unit in a shared curriculum.
Over the last few months I’ve been exploring and detailing a provisional binding of the InLOC spec to JSON-LD (spec; site). My conclusion is that JSON is better matched to linked data than XML is, if you understand how to structure JSON in the JSON-LD way. Here are my reflections, which I hope add something to the JSON-LD official documentation.
A while back I summarised the input about semantics and academic coding that Lorna and I had made on behalf of Cetis for a study on possible reforms to JACS, the Joint Academic Coding System. That study has now been published.
JACS is mainatained by HESA (the Higher Education Statistics Agency) and UCAS (Universities and Colleges Admissions Service) as a means of classifying UK University courses by subject; it is also used by a number of other organisations for classification of other resources, for example teaching and learning resources. The report (with appendices) considers the varying requirements and uses of subject coding in HE and sets out options for the development of a replacement for JACS.
Of course, this is all only of glancing interest, until you realise that stuff like Unistats and the Key Information Set (KIS) are powered by JACS.
- See more
The Learning Resource Metadata Initiative aimed to help people discover useful learning resources by adding to the schema.org ontology properties to describe educational characteristics of creative works. Well, as of the release of schema draft version 1.0a a couple of weeks ago, the LRMI properties are in the official schema.org ontology.
Schema.org represents two things: 1, an ontology for describing resources on the web, with a hierarchical set of resource types each with defined properties that relate to their characteristics and relationships with other things in the schema hierarchy; and 2, a syntax for embedding these into HTML pages–well, two syntaxes, microdata and RDFa lite. The important factor in schema.org is that it is backed by Google, Yahoo, Bing and Yandex, which should be useful for resource discovery. The inclusion of the LRMI properties means that you can now use schema.org to mark up your descriptions of the following characteristics of a creative work:
audience the educational audience for whom the resource was created, who might have educational roles such as teacher, learner, parent.
educational alignment an alignment to an established educational framework, for example a curriculum or frameworks of educational levels or competencies. Expressed through an abstract thing called an Alignment Object which allows a link to and description of the node in the framework to which the resource aligns, and specifies the nature of the alignment, which might be that the resource ‘assesses’, ‘teaches’ or ‘requires’ the knowledge/skills/competency to which the resource aligns or that it has the ‘textComplexity’, ‘readingLevel’, ‘educationalSubject’ or ‘educationLevel’ expressed by that node in the educational framework.
educational use a text description of purpose of the resource in education, for example assignment, group work.
interactivity type The predominant mode of learning supported by the learning resource. Acceptable values are ‘active’, ‘expositive’, or ‘mixed’.
is based on url A resource that was used in the creation of this resource. Useful for when a learning resource is a derivative of some other resource.
learning resource type The predominant type or kind characterizing the learning resource. For example, ‘presentation’, ‘handout’.
time required Approximate or typical time it takes to work with or through this learning resource for the typical intended target audience
typical age range The typical range of ages the content’s intended end user.
Of course, much of the other information one would want to provide about a learning resource (what it is about, who wrote it, who published it, when it was written/published, where it is available, what it costs) was already in schema.org.
Unfortunately one really important property suggested by LRMI hasn’t yet made the cut, that is useRightsURL, a link to the licence under which the resource may be used, for example the creative common licence under which is has been released. This was held back because of obvious overlaps with non-educational resources. The managers of schema.org want to make sure that there is a single solution that works across all domains.
To promote the uptake of these properties, the Association of Educational Publishers has released two new user guides.
The Smart Publisher’s Guide to LRMI Tagging (pdf)
The Content Developer’s Guide to the LRMI and Learning Registry (pdf)
There is also the InBloom Tagger described and demonstrated in this video.
As the last two resources show, LRMI metadata is used by the Learning Registry and services built on it. For what it is worth, I am not sure that is a great example of its potential. For me the strong point of LRMI/schema.org is that it allows resource descriptions in human readable web pages to be interpreted as machine readable metadata, helping create services to find those pages; crucially the metadata is embedded in the web page in way that Google trusts because the values of the metadata are displayed to users. Take away the embedding in human readable pages, which is what seems to happen when used with the learning registry, and I am not sure there is much of an advantage for LRMI compared to other metadata schema,–though to be fair I’m not sure that there is any comparative disadvantage either, and the effect on uptake will be positive for both sides. Of course the Learning Registry is metadata agnostic, so having LRMI/schema.org metadata in there won’t get in the way of using other metadata schema.
I was lucky enough to be on the LRMI technical working group that helped make this happen. It makes me vary happy to see this progress.
Lorna and I recently contributed a study on possible reforms to JACS, a study which is part of a larger piece of work on Redesigning the HE data landscape. JACS, the Joint Academic Coding System, is mainatained by HESA (the Higher Education Statistics Agency) and UCAS (Universities and Colleges Admissions Service) as a means of classifying UK University courses by subject; it is also used by a number of other organisations for classification of other resources, for example teaching and learning resources. The study to which we were contributing our thoughts had already identified a problem with different people using JACS in different ways, which prompted the first part of this post. We were keen to promote technical changes to the way that JACS is managed that would make it easier for other people to use (and incidentally might help solve some of the problems in further developing JACS for use by HESA and UCAS), which are outline in the second part.
There’s nothing new here, I’m posting these thoughts here just so that they don’t get completely lost.
One of the issue identified with the use of JACS is that “although ostensibly believing themselves to be using a single system of classification, stakeholders are actually applying JACS for a variety of different purposes” including Universities who “often try to align JACS codes to their cost centres rather than adopting a strictly subject-based approach”. The cost centres in question are academic schools or departments, which are discipline based. This is problematic to the use of JACS to monitor which subjects are being learnt since the same subject may be taught in several departments. A good example of this is statistics, which is taught across many fields from Mathematics through to social sciences, but there are many other examples: languages taught in mediaeval studies and business translation courses, elements of computing taught in electronic engineering and computer science and so on. One approach would be to ignore the discipline dimension, to say the subject is the same regardless of the different disciplinary slants taken, that is to say statistics taught to mathematicians is the same as statistics taught to physicists is the same as statistics taught to social sciences. This may be true at a very superficial level, but obviously the relevance of theoretical versus practical elements will vary between those disciplines, as will the nature of the data to be analysed (typically a physicist will design an experiment to control each variable independently so as not to deal with multivariate data, this is not often possible in social sciences and so multivariate analysis is far more important). When it comes to teaching and learning resources something aimed at one discipline is likely to contain examples or use an approach not suited to others.
Perhaps more important is that academics identify with a discipline as being more than a collection of subjects being taught. It encapsulates a way of thinking, a framework for deciding on which problems are worth studying and a way of approaching these problems. A discipline is a community, and an academic who has grown up in a community will likely have acquired that community’s view of the subjects important to it. This should be taken into account when designing a coding scheme that is to be used by academics since any perception that the topic they teach is being placed under someone else’s discipline will be resisted as misrepresenting what is actually being taught, indeed as a threat to the integrity of the discipline.
More objectively, the case for different disciplinary slants on a problem space being important is demonstrated by the importance of multidisciplinary approaches to solving many problems. Both the reductionist approach of physics and the holistic approach of humanities and social sciences have their strengths, and it would be a shame if the distinction were lost.
The ideal coding scheme would be able to represent both the subject learnt and the discipline context in which it was learnt.
Tim Berners-Lee suggested a 5 star deployment scheme for open data on the world wide web:
* make your stuff available on the Web (whatever format) under an open licence
** make it available as structured data (e.g., Excel instead of image scan of a table)
*** use non-proprietary formats (e.g., CSV instead of Excel)
**** use URIs to denote things, so that people can point at your stuff
**** link your data to other data to provide context
Currently JACS fails to meet the open licence requirement for 1-star data explicitly, but that seems to be a simple omission of a licensing statement that shows the intention that JACS should be freely available for others to use. It is important that this is fixed, but aside from this, JACS operates at about 3-star level. Assigning URIs to JACS subjects and providing useful information when someone accesses these URIs will allow JACS to be part of the web of linked open data. The benefits of linking data over the web include:
In practical terms, implementing this would mean:
The main overhead is in setting up, maintaining and managing the data provided by the web service, but Southampton University have already set one up for their own use. (The only problem with the Southampton service–and I believe Oxford have done something similar–is a lack of authority, i.e. it isn’t clear to other users whether the data from this service is authoritative, up to date, used under a correct license, sustainable.)
SKOS (Simple Knowledge Organization System) is a semantic web application of RDF which provides a model for expressing the basic structure and content of concept schemes such as thesauri, classification schemes, subject heading lists, taxonomies, folksonomies, and other similar types of controlled vocabulary. It allows for the description of a concept and the expression of the relationship betweens pairs of concepts. But first the concept must be identified as such, with a URI. For example:
jacs:H713 rdf:type skos:concept
In this example jacs: is shorthand for the JACS base URI, http://id.jacs.ac.uk/ as suggested above; rdf: and skos: are shorthand for the base URIs for RDF and SKOS. This triple says “The thing identified by http://id.jacs.ac.uk/H713 is a resource of type (as defined by RDF) concept (as defined by SKOS)”.
Other assertions can be made about the resource, e.g. the preferred label to be used for it and a scope note for it.
jacs:H713 skos:prefLabel “Production Processes”
jacs:H713 skos:scopeNote “The study of the principles of engineering as they apply to efficient application of production-line technology.”
Assuming the other JACS codes have been suitably identified, relationships between them can be described:
jacs:H713 skos:broader jacs:H710
jacs:H713 skos:related jacs:H830
Once JACS is on the semantic web relationships between the JACS subjects and things in other schemas can also be represented
http://example.org/123 dct:subject jacs:H713
(The resource identified by the URI http://example.org/123 is about the subject identified by jacs:H713).
Last weekend, a motley crew of designers, students, developers, business and government people came together in Edinburgh to prototype designs and apps to help learners manage their journeys. With help, I built a prototype that showed how curriculum and course offering data can be combined with e-portfolios to help learners find their way.
The first official Scottish government data jam, facilitated by Snook and supported by TechCube, is part of a wider project to help people navigate the various education and employment options in life, particularly post 16. The jam was meant to provide a way to quickly prototype a wide range of ideas around the learner journey theme.
While many other teams at the jam built things like a prototype social network, or great visualisations to help guide learners through their options, we decided to use the data that was provided to help see what an infrastructure could look like that supported the apps the others were building.
In a nutshell, I wanted to see whether a mash-up of open data in open standard formats could help answer questions like:
Here’s a slide deck that outlines the results. For those interested in the nuts and bolts read on to learn more about how we got there.
Where is the learner?
To show how you can map where someone is on their learning journey, I made up an e-portfolio. Following an excellent suggestion by Lizzy Brotherstone of the Scottish Government, I nicked a story about ‘Ryan’ from an Education Scotland website on learner journeys. I recorded his journey in a Mahara e-portfolio, because it outputs data in the standard LEAP2a format- I could have used PebblePad as well for the same reason.
I then transformed the LEAP2a XML into very rough but usable RDF using a basic stylesheet I made earlier. Why RDF? Because it makes it easy for me to mash up the portfolios with other datasets; other data formats would also work. The made-up curriculum identifiers were added manually to the RDF, but could easily have been taken from the LEAP2a XML with a bit more time.
Where can we suggest they go next?
I expected that the Curriculum for Excellence would provide the basic structure to guide Ryan from his school qualifications to a college course. Not so, or at least, not entirely. The Scottish Qualifications Framework gives a good idea of how courses relate in terms of levels (i.e. from basic to a PhD and everything in between), but there’s little to join subjects. After a day of head scratching, I decided to match courses to Ryan’s qualifications by level and comparing the text of titles. We ought to be able to do better than that!
The course data set was provided to us was a mixture of course descriptions from the Scottish Qualifications Authority, and actual running courses offered by Scottish colleges all in one CSV file. During the jam, Devon Walshe of TechCube made a very comprehensive data set of all courses that you should check out, but too late for me. I had a brief look at using XCRI feeds like the ones from Adam Smith college too, but went with the original CSV in the end. I tried using LOD Refine to convert the CSV to RDF, but it got stuck on editing the RDF harness for some reason. Fortunately, the main OpenRefine version of the same tool worked its usual magic, and four made-up SQA URIs later, we were in business.
This query takes the email of Ryan as a unique identifier, then finds his qualification subjects and level. That’s compared to all courses from the data jam course data set, and whittled down to those courses that match Ryan’s qualifications and are above the level he already has.
The result: too many hits, including ones that are in subjects that he’s unlikely to be interested in.
So let’s throw in his interests as well. Result: two courses that are ideal for Ryan’s skills, but are a little above his level. So we find out all the sensible courses that can take him to his goal.
What can help them get there?
One other quirk about the curriculum for excellence appears to be that there are subject taxonomies, but they differ per level. Intralect implemented a very nice one that can be used to tag resources up to level 3 (we think). So Intralect’s Janek exported the vocabulary in two CSV files, which I imported in my triple store. He then built a little web service in a few hours that takes the outcome of this query, and returns a list of all relevant resources in the Intralibrary digital repository for stuff that Ryan has already learned, but may want to revisit.
Who can help or inspire them?
It’s always easier to have someone along for the journey, or to ask someone who’s been before you. That’s why I made a second e-portfolio for Paula. Paula is a year older than Ryan, is from a different, but nearby school, and has done the same qualifications. She’s picked the same qualification as a goal that we suggested to Ryan, and has entered it as a goal on her e-portfolio. Ryan can get it touch with her over email.
This query takes the course suggested to Ryan, and matches it someone else’s stated academic goal, and reports on what she’s done, what school she’s from, and her contact details.
Conclusion
For those parts of the Curriculum for Excellence for which experiences and outcomes have been defined, it’d be very easy to be very precise about progression, future options, and what resources would be particularly helpful for a particular learner at a particular part of the journey. For the crucial post 16 years, this is not really possible in the same way right now, though it’s arguable that its all the more important to have solid guidance at that stage.
Some judicious information architecture would make a lot more possible without necessarily changing the syllabus across the board. Just a model that connects subject areas across the levels, and school and college tracks would make more robust learner journey guidance possible. Statements that clarify which course is an absolute pre-requisite for another, and which are suggested as likely or preferable would make it better still.
We have the beginnings of a map for learner journeys, but we’re not there yet.
Other than that, I think agreed identifiers and data formats for curriculum parts, electronic portfolios or transcripts and course offerings can enable a whole range of powerful apps of the type that others at the data jam built, and more. Thanks to standards, we can do that without having to rely on a single source of truth or a massive system that is a single point of failure.
Find out all about the other great hacks on the learner journey data jam website.
All the data and bits of code I used are available on github
Like most places, the University of Bolton keeps its data in many stores. That’s inevitable with multiple systems, but it makes getting a complete picture of courses and students difficult. We test an approach that promises to integrate all this data, and some more, quickly and cheaply.
Integrating a load of data in a specialised tool or data warehouse is not new, and many institutions have been using them for a while. What Bolton is trying in its JISC sponsored course data project is to see whether such a warehouse can be built out of Linked Data components. Using such tools promises three major advantages over existing data warehouse technology:
It expects data to be messy, and it expects it to change. As a consequence, adding new data sources, or coping with changes in data sources, or generating new reports or queries should not be a big deal. There are no schemas to break, so no major re-engineering required.
It is built on the same technology as the emergent web of data. Which means that increasing numbers of datasets – particularly from the UK government – should be easily thrown into the mix to answer bigger questions, and public excerpts from Bolton’s data should be easy to contribute back.
It is standards based. At every step from extracting the data, transforming it and loading it to querying, analysing and visualising it, there’s a choice of open and closed source tools. If one turns out not to be up to the job, we should be able to slot another in.
But we did spend a day kicking the tires, and making some initial choices. Since the project is just to pilot a Linked Enterprise Data (LED) approach, we’ve limited ourselves to evaluate just open source tools. We know there plenty of good closed source options in any of the following areas, but we’re going to test the whole approach before deciding on committing to license fees.
Data sources
Before we can mash, query and visualise, we need to do some data extraction from the sources, and we’ve come down on two tools for that: Google Refine and D2RQ. They do slightly different jobs.
Refine is Google’s power tool for anyone who has to deal with malformed data, or who just wants to transform or excerpt from format to another. It takes in CSV or output from a range of APIs, and puts it in table form. In that table form, you can perform a wide range of transformations on the data, and then export in a range of formats. The plug-in from DERI Galway, allows you to specify exactly how the RDF – the linked data format, and heart of the approach – should look when exported.
What Refine doesn’t really do (yet?) is transform data automatically, as a piece of middleware. All your operations are saved as a script that can be re-applied, but it won’t re-apply the operations entirely automagically. D2RQ does do that, and works more like middleware.
Although I’ve known D2RQ for a couple of years, it still looks like magic to me: you download, unzip it, tell it where your common or garden relational database is, and what username and password it can use to get in. It’ll go off, inspect the contents of the database, and come back with a mapping of the contents to RDF. Then start the server that comes with it, and the relational database can be browsed and queried like any other Linked Data source.
Since practically all relevant data in Bolton are in a range of relational databases, we’re expecting to use D2R to create RDF data dumps that will be imported into the data warehouse via a script. For a quick start, though, we’ve already made some transforms with Refine. We might also use scripts such as Oxford’s XCRI XML to RDF transform.
Storage, querying and visualisation
We expected to pick different tools for each of these functions, but ended up choosing one, that does it all- after a fashion. Callimachus is designed specifically for rapid development of LED applications, and the standard download includes a version of the Sesame triplestore (or RDF database) for storage. Other triple stores can also be used with Callimachus, but Sesame was on the list anyway, so we’ll see how far that takes us.
Callimachus itself is more of a web application on top that allows quick visualisations of data excerpts- be they straight records of one dataset or a collection of data about one thing from multiple sets. The queries that power the Callimachus visualisations have limitations – compared to the full power of SPARQL, the linked data query language – but are good enough to knock up some pages quickly. For the more involved visualisations, Callimachus SPARQL 1.1 implementation allows the results a query to be put out as common or garden JSON, for which many different tools exist.
Next steps
We’ve made some templates already that pull together course information from a variety of sources, on which I’ll report later. While that’s going on, the main other task will be to set up the processes of extracting data from the relational databases using D2R, and then loading it into Callimachus using timed scripts.